Does the agency still require to employ a large contingent of data scientists to build machine learning models? Or does AutoML reduce the demand for this evasive talent? Let’s read this blog to understand anything about AutoML and how to fill in the AI talent strategy gaps.
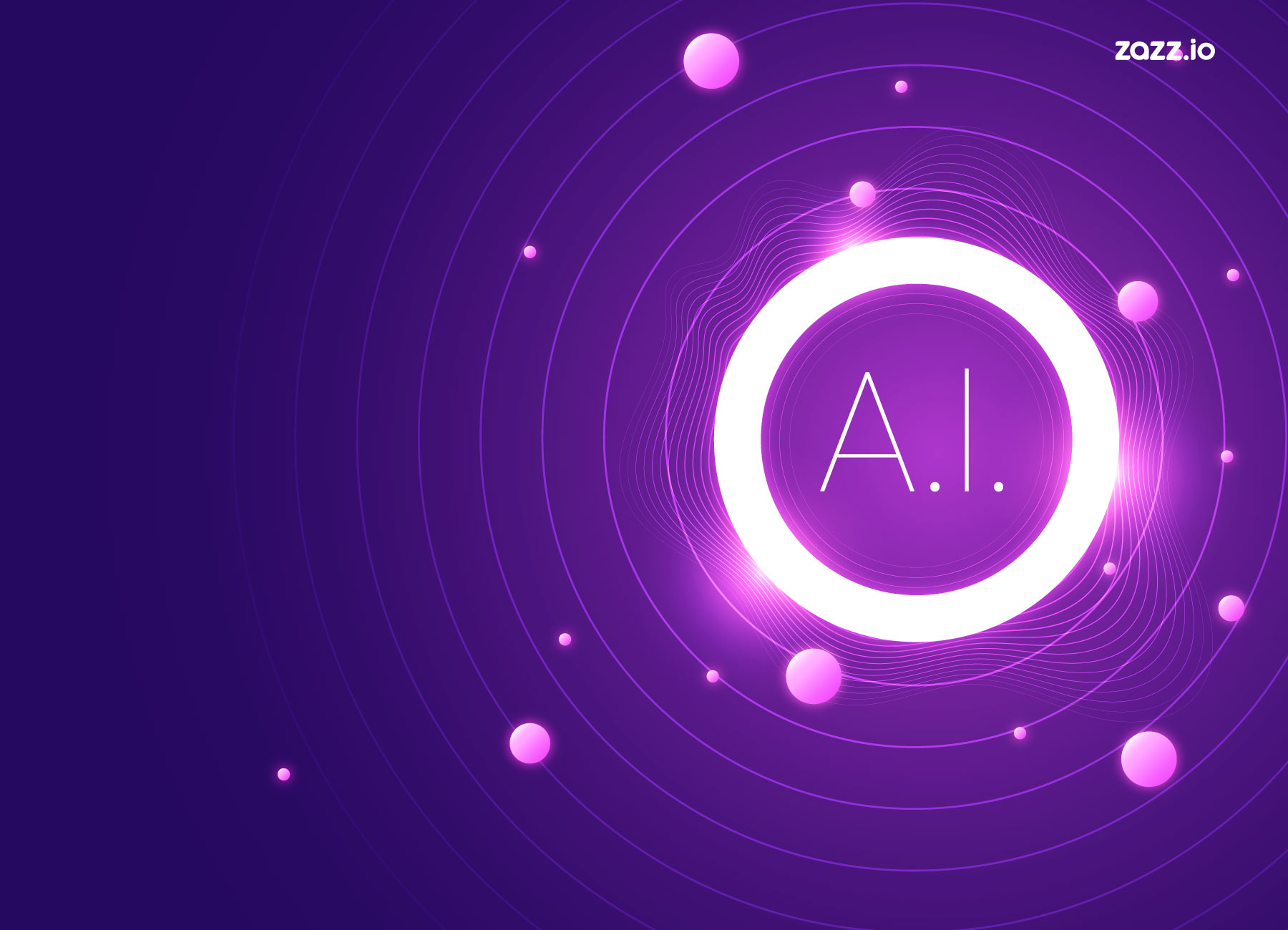
Glimpse on Artificial Intelligence Talent Strategy Gap
AI experts are expensive, with reported average annual salaries. But before you even worry about hiring an AI expert, you need to find one. As per a study, there are only 22,000 qualified computer scientists worldwide capable of building AI systems. Of these, only 3,000 are looking for work, and it is estimated that there are 10,000 positions currently available for artificial intelligence experts in the US alone.
Too few scientists have the knowledge or experience needed to work in this industry. Still, because Artificial Intelligence Application Development Companies are developing so fast, it is difficult for academic institutions to keep up with companies’ changing needs and provide graduates with the skills needed.
As more companies, sectors, and markets branch into AIartificial intelligence-related technologies, the gap between the needed and available personnel is widening, and the need for other solutions becomes more pressing. Small, unqualified, or overwhelmed development teams are more likely to make mistakes, try and inappropriately apply existing models to new data, or miss problems with data. All of these problems can cause significant damage to the developed system.
What is Automated Machine Learning – AutoML?
AutoML is a generic expression to denote a piece of software that automatically performs Machine Learning tasks. They usually automate all pipeline processing such as, for example, cleaning, encoding, feature and model selection, and hyperparameter tuning. Such software can be Python libraries such as Auto-Sklearn or software programs such as Robot Data.
AutoML software replaces all the tedious steps that require more time for a Data Scientist’s job. They make all combinations of multiple channel parameters and select the best combination that maximizes multiple performance metrics in k-fold cross-validation using multiple search algorithms.
They can simplify the life of someone who has to build a model from scratch, and sometimes they explore combinations and scenarios that a Data Scientist might not have thought of.
Data Science is Not Just Machine Learning
A Data Scientist analyzes the hidden information in the data, extracts useful correlations, provides assistance to prepare the correct data to feed the ML pipeline. And provides useful insight into businesses that have created their data. These things are the most important parts of Data Science and cannot be completely automated. They rely on in-depth knowledge of business, on the strong and effective use of business language that people speak, and more than anything else, on which business managers speak.
All of these things make a Data Scientist’s job more complex and exciting than running a Machine Learning model, and that is beyond the scope of AutoML.
AutoML software automates Machine Learning tasks, not the entire Data Science process. Machine Learning is only a small part of the Data Scientist’s job and perhaps not the most important or the most challenging. Moreover, understanding data, information, and the business context is a real challenge for a Data Scientist. If this task is not fully completed, Machine Learning will never be a magic wand that solves all problems.
AutoML Does Not Work Alone
AutoML is software, so it always takes someone with the right skills to use it. Professional Data Scientists must validate AutoML results to ensure they are correct and make sense in a manufactured business environment. It’s not uncommon to come up with a model that looks perfect on paper.
But it doesn’t yield any useful business insights or, in the worst case, the predictions are just trivial. That is the reason a Data Scientist has to be around to ensure that the model is stating something fresh and not just chewing on something old.
How Does AutoML Fill The Artificial Intelligence Talent Strategy Gap?
AutoML enables smaller, overwhelmed, or understaffed companies to achieve a level of analytical sophistication. Apart from the distinct advantages on time and in essence, using AutoML also reduces the room for error.
AutoML products guide users by creating and using new algorithms in easy-to-use and comprehensive settings, minimizing code errors or misapplications. Also, they permit more personnel to use ML because the entry barrier for AutoML products is low as compared to artificial intelligence.
With a user-driven interface, even novice developers can quickly create useful technology. And it saves time and increases productivity because less energy is spent building a lot of institutional knowledge.
The Future of AutoML
Essentially, AutoML’s goal is to automate repetitive tasks like pipeline creation and hyperparameter set up so that data scientists can spend more of their time-solving business problems.
AutoML also aims to make the technology available to everyone rather than a select few. However, machine learning app development agencies say AutoML and data scientists can work together to speed up the ML process so that real machine learning effectiveness can be used.
The success or failure of AutoML depends on the adoption and progress made in this sector. However, AutoML is a big part of machine learning in the future.
Final Words
Zazz thinks Data Scientists need to keep up with changes and innovations, so AutoML can be a handy companion if they start using it properly. If they automate tedious tasks, they will likely have more time to spend analyzing information.
Moreover, it is the primary and real goal of a Data Scientist. After reading the complete article, you encounter any query related to this topic. So, let’s discuss with our respected experts; they will help you immediately.
Recent Articles
Table of Content 1. What is IT Staff Augmentation? 2....
Table of Content 1. What is Staff Augmentation for App...
Table of Content 1. What is IT Staff Augmentation? 2....